Top AI Inventory Management Software Features Every Aviation Supply Chain Manager Needs
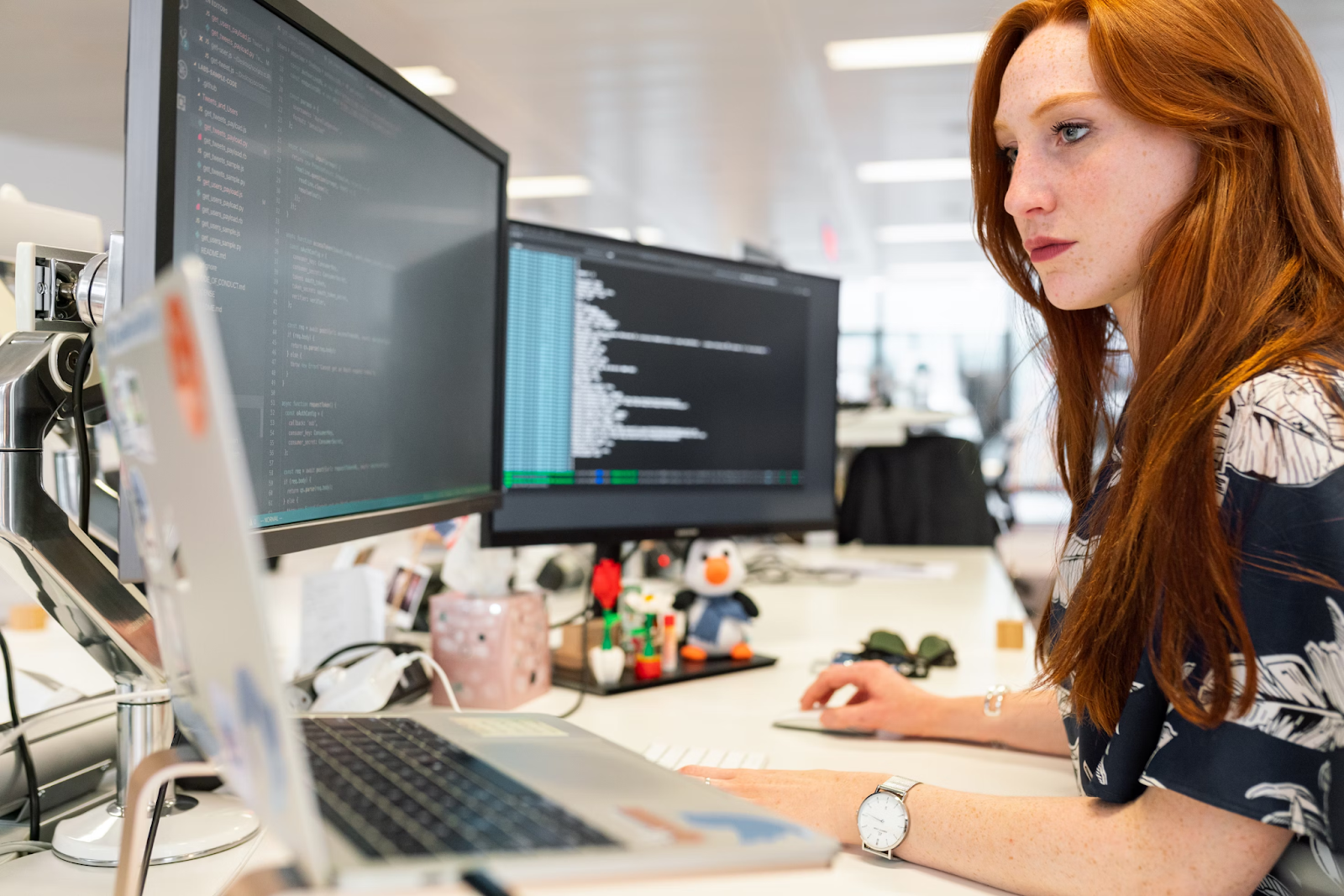
Amid a bustling air travel scene, the FAA oversees over 45,000 flights every single day, transporting nearly 3 million passengers across 29 million square miles of air space (FAA: Air Traffic Control by the Numbers).
Coordinating safe travel for this level of traffic requires the precise coordination of maintenance schedules, part availability, and regulatory compliance. Even minor inefficiencies in inventory management can ripple into significant operational challenges, grounding aircraft and causing cascading delays.
In the aviation industry, an Aircraft on Ground (AOG) event can cost airlines up to $10,000 per hour, encompassing expenses such as maintenance, passenger accommodations, and lost revenue.
The substantial costs underscore the critical importance of efficient inventory management. Delays in procuring essential components can lead to prolonged aircraft downtime, directly impacting an airline's operational efficiency and profitability.
Traditional inventory management systems often struggle with the complexities of global supply chains, specialized aircraft parts, and stringent regulatory requirements. This is where AI-driven inventory management solutions come into play.
Leveraging artificial intelligence, these systems provide predictive analytics, real-time tracking, and other automated processes, enabling aviation supply chain managers to maintain optimal inventory levels and timely availability of critical components.
In this article, we will explore the essential features of AI in inventory management software and how it can significantly benefit aviation supply chain operations.
Predictive analytics for demand forecasting
Predictive analytics is a cornerstone of AI inventory management software, offering supply chain managers the ability to make data-driven decisions with confidence and precision. Using advanced machine learning algorithms, AI systems analyze historical data, real-time operational factors, and evolving trends to forecast demand for critical components.
For aviation supply chain managers, this means anticipating needs more effectively, reducing the risk of stockouts, and avoiding costly overstocking.
Predictive models can account for factors like maintenance schedules, fleet utilization, and external disruptions such as weather patterns or geopolitical events to adjust forecasts.
The FAA's adoption of AI-driven predictive analytics, such as in the Aircraft Collision Avoidance System (ACAS), showcases the transformative potential of these tools to identify risks and proactively implement solutions.
By integrating predictive insights into inventory management, aviation companies can align their resources with operational needs and ensure that critical components are available whenever and wherever they are required. This level of precision streamlines operations and drives significant cost savings across the supply chain (Aviation Maintenance Magazine).
Real-time inventory tracking
AI inventory management systems bring unprecedented speed and accuracy to a traditionally tedious process. This is a critical win for aviation supply chains where delays in locating components can cascade into costly operational disruptions. AI-powered systems rely on a combination of IoT sensors and ERP platforms to monitor and manage inventory movement with exacting accuracy.
IoT sensors installed on inventory items or within storage facilities provide continuous updates on the location and condition of parts. For example, RFID tags and GPS-enabled devices transmit data about where specific components are stored, how they are transported, and whether environmental conditions, such as temperature or humidity, meet regulatory standards. This constant stream of data reduces reliance on manual tracking, which is prone to errors and delays.
Enterprise resource planning (ERP) platforms can consolidate the data collected by IoT sensors into a centralized dashboard. This integration gives supply chain managers a comprehensive view of inventory across multiple locations. For instance, managers can access real-time updates on stock levels, identify parts approaching expiration dates, or locate critical components required for time-sensitive repairs.
Instead of reacting to shortages or surpluses after they occur, these systems enable proactive decision-making to avoid them. A supply chain manager using real-time tracking tools can identify a regional warehouse experiencing low stock levels and immediately reallocate inventory or reorder parts before a disruption occurs. Delivering such actionable insights, AI-powered tracking keeps fleets operational.
Dynamic reordering systems
Static reorder points in traditional inventory systems often fail to account for sudden shifts in demand or unexpected supply chain disruptions, leaving gaps that can cascade into operational inefficiencies. AI solutions address these limitations with dynamic reordering capabilities that adapt to real-time conditions. These conditions include fluctuating demand patterns influenced by seasonal trends, sudden spikes due to unforeseen maintenance needs, supplier delays caused by logistical issues, and external factors such as adverse weather or geopolitical events that disrupt supply chains.
Real-time data analysis drives these systems, incorporating historical trends, supplier performance metrics, and operational schedules to recalibrate reorder points with exceptional precision. Inventory levels remain optimized, reducing the risks of stockouts while avoiding unnecessary overstocking. Adaptive capabilities like these enable aviation supply chain managers to proactively respond to shifting conditions, ensuring smooth operations even in unpredictable scenarios.
These systems integrate historical data, real-time demand signals, and supplier performance metrics to recalibrate reorder points dynamically (Aviation Maintenance Magazine). AI models can factor in maintenance schedules, part failure trends, and external influences like weather disruptions or geopolitical events to anticipate inventory needs more accurately. Through leveraging predictive insights, dynamic reordering ensures the availability of critical parts.
Additionally, AI-powered systems optimize order timing and quantities by analyzing supplier lead times and pricing fluctuations. If a supplier’s delivery times increase due to regional disruptions, the system adjusts reorder schedules preemptively. This anticipatory approach reduces carrying costs, prevents stockouts, and enhances overall supply chain resilience.
Supplier performance analysis
AI inventory management software also transforms the way aviation companies evaluate and collaborate with suppliers. Here’s how:
Identifying top-performing suppliers
AI systems analyze a range of key performance indicators (KPIs), such as supplier lead times, delivery accuracy, and defect rates. A supplier consistently meeting 99% on-time delivery rates could be flagged as a preferred vendor, allowing supply chain managers to prioritize contracts with them. Conversely, suppliers with frequent delays or inconsistent order quality are quickly identified as risks.
This granular evaluation empowers supply chain managers to shift from reactive problem-solving to proactive supplier selection, ensuring that high-performing suppliers are objectively prioritized for critical components.
Improving contract negotiations
Aggregating and analyzing pricing trends and order fulfillment data allows AI to give aviation companies a detailed understanding of market benchmarks. For example, AI can identify discrepancies such as a supplier's price increase without corresponding service improvements, equipping managers with valuable insights to renegotiate contracts or explore alternative providers.
This data-driven negotiation approach reduces procurement costs while holding suppliers accountable.
Mitigating supply chain disruptions
AI systems flag early warning signs, such as a supplier's lengthening lead times or declining order accuracy, enabling companies to take preemptive action. For example, if geopolitical events disrupt a supplier’s operations, the system can recommend alternative suppliers with comparable delivery capabilities based on historical data.
Proactively mitigating risks minimizes delays and prevents cascading operational disruptions
Enhancing long-term collaboration
Tracking supplier performance trends over time, AI fosters a partnership-oriented approach. Data revealing consistent improvements in delivery accuracy or reduced defect rates could encourage aviation companies to offer long-term contracts or collaborative incentives, such as shared inventory data or co-investments in quality improvement programs.
Such a collaborative approach builds a more resilient supply chain, positioning aviation companies to better navigate market fluctuations and demand spikes.
Pinpointing inefficiencies
AI enables a granular view of when and where inefficiencies arise, such as suppliers consistently failing to meet contractually agreed performance metrics. Adding these insights into supplier scorecards, managers can decide whether to initiate corrective action, renegotiate terms, or terminate underperforming relationships.
These targeted strategies for vendor inefficiency save time and money, ensuring supply chains run with minimal friction.
Inventory categorization with machine learning
Aviation supply chains are uniquely complex, with inventory ranging from everyday consumables like lubricants and filters to rare, high-value parts like turbine blades or avionics components.
Categorizing inventory often relies on manual processes, which are time-consuming and error-prone, leading to stockouts and other inefficiencies. Machine learning (ML) transforms the process by automating inventory categorization and ensuring that aviation companies can categorize goods accurately.
How machine learning enhances inventory categorization
- Data analysis across multiple dimensions: Machine learning algorithms can process vast datasets, analyzing factors like usage patterns, part criticality, demand variability, and historical maintenance data.
- Dynamic categorization: Unlike static classification systems, ML-based tools dynamically update inventory categories in real-time. For instance, a component initially classified as slow-moving may shift to high-priority if sudden demand arises due to fleet upgrades or regulatory changes.
- Critical component identification ML tools can flag components essential for aircraft safety and operation, ensuring these are always well-stocked. For example, parts critical to Airworthiness Directives (ADs) can be tagged as high-priority items, preventing compliance delays or safety risks.
Benefits of machine learning in inventory categorization
Machine learning transforms inventory categorization in aviation by enhancing efficiency and precision. It helps supply chain managers allocate resources to high-priority and frequently used components, cutting waste from overstocking less critical items.
ML also tracks demand trends and lifecycle data, flagging parts nearing obsolescence so managers can phase them out and plan replacements before disruptions.
Finally, dynamic updates and real-time visibility into inventory categories streamline operations, reducing the need for manual oversight. Teams can shift focus from spreadsheet entry to strategic initiatives, increasing overall operational efficiency and enabling aviation companies to adapt quickly to changing demands.
Integration with ERP systems
For AI inventory management software to deliver maximum value, it must integrate deeply with existing ERP systems. This integration goes beyond surface-level compatibility, involving advanced data exchange protocols, API connectivity, and system alignment to ensure the AI platform operates as a seamless extension of the ERP environment.
How integration happens
1. API and middleware connections
AI systems utilize APIs (Application Programming Interfaces) and middleware to establish two-way communication with ERP platforms like SAP, Oracle NetSuite, or Microsoft Dynamics. This ensures that data from procurement, inventory, maintenance, and finance modules is not only shared but also processed and analyzed in real-time.
2. Data synchronization
Integration pipelines continuously sync data between AI inventory systems and ERP modules, eliminating delays. Inventory updates like new receipts or dispatches are instantly reflected to refine demand forecasts. Maintenance schedules can be added to trigger AI recommendations for required components and stock levels.
3. Automated workflows
The integration enables workflows to span across systems. A maintenance alert from the ERP can automatically prompt AI to evaluate stock levels and trigger a reorder if necessary. Procurement modules in the ERP automatically incorporate insights from AI-driven supplier performance analysis, ensuring smarter purchasing decisions.
4. Unified dashboards
Consolidate ERP data and AI insights into centralized dashboards. Managers can review inventory health, supplier performance, and cost projections from within their existing ERP interface.
5. Error reduction through real-time validation
Integrated systems validate data inputs across platforms to ensure consistency and accuracy. For example, if the AI detects a discrepancy in stock levels between its analysis and the ERP database, it flags the issue for immediate resolution.
Compliance-related data, such as part certifications or expiry dates, are cross-checked against regulatory requirements stored in ERP modules.
6. Custom configuration
Integration is tailored to the specific workflows and data architecture of the aviation company. This may involve customizing APIs, defining unique data hierarchies, or setting rules for how information flows between the AI system and the ERP.
Cost optimization
AI-driven inventory management software revolutionizes cost control for aviation companies by addressing inefficiencies across the supply chain.
- Reducing carrying costs: AI analyzes stock levels against demand forecasts, identifying excess inventory while maintaining critical buffer stock.
- Minimizing waste: Tracks part lifecycles to ensure components are used before expiration, reducing losses from obsolete inventory. Flags underutilized items, enabling supply chain managers to adjust procurement strategies.
- Improving supplier cost efficiency: Analyzes market trends to recommend optimal procurement timing, particularly for high-value parts with volatile pricing. Highlights suppliers with the best cost-performance ratio for efficient budget allocation.
- Operational cost savings: Automates inventory audits and demand forecasting, reducing administrative overhead for managing fleets across multiple locations. Prevents costly last-minute purchases by ensuring accurate stock tracking.
- Proactive cost management: Centralized dashboards provide real-time insights into cost drivers, enabling targeted interventions to reduce unnecessary expenses.
The future of AI inventory management in aviation
AI and other emerging technologies are poised to take on an even greater role in inventory management as the aviation industry faces growing complexities.
Autonomous inventory systems powered by robotics
The integration of AI with robotics is paving the way for autonomous inventory management systems capable of handling everything from stocking to replenishment. These systems leverage machine learning to prioritize tasks and coordinate with robotics for precise execution.
For example, automated guided vehicles (AGVs) and robotic arms could streamline the handling of delicate, high-value parts like auxiliary power units (APUs) or turbine blades, reducing human error and labor costs.
Blockchain for end-to-end transparency
Blockchain technology can bring transparency to supply chains by creating an immutable ledger for all inventory transactions. This ensures every part's journey—from supplier to aircraft—is thoroughly documented, reducing risks associated with counterfeit or uncertified components.
Blockchain’s traceability also simplifies compliance audits by providing regulators with a verifiable digital record of parts' certifications and handling.
For instance, a blockchain-integrated system provides an immutable audit trail that securely records the chain of custody for critical components. This ensures every transaction—from manufacturing to installation—is documented and tamper-proof, offering unparalleled traceability.
While blockchain doesn’t analyze or interpret data, its ability to preserve an unalterable record complements AI systems that piece together and analyze this information. By leveraging blockchain’s transparency and security, aviation companies can confidently confirm a critical component’s compliance with FAA regulations, streamlining the inspection process and reducing risks associated with data discrepancies.
Collaborative platforms for synchronized supply chains
AI-driven collaborative platforms could enable real-time data sharing among stakeholders, including airlines, MRO providers, and manufacturers.
These platforms can use predictive analytics to synchronize inventory needs across the supply chain, preventing bottlenecks and ensuring parts availability. In an AI ecosystem, automated alerts to suppliers would replenish inventory based on an airline’s predictive maintenance schedules, reducing downtime across fleets.
Hyper-personalized inventory strategies through AI
Advanced AI models will shift inventory management from reactive to hyper-personalized. Able to analyze the most granular of data points with ease, AI can spot usage patterns that humans would miss and then craft tailored recommendations for individual fleets or specific aircraft models.
Digital twins and simulation-based planning
The adoption of digital twin technology will allow aviation companies to simulate inventory scenarios before making decisions. Using real-time data, these virtual replicas of supply chains can test the impact of inventory adjustments—such as shifting parts between warehouses or changing reorder points—without disrupting actual operations.
Digital twins could also help companies model the effects of extreme weather and other external events on their supply chains.
AI-powered risk assessment tools
Future AI systems will feature enhanced risk assessment tools able to identify vulnerabilities across interconnected supply chains.
These systems will use neural networks to evaluate not just supplier performance but also external risks, such as geopolitical instability or raw material shortages. Proactively addressing these risks gives aviation companies a competitive edge by ensuring continuity while others are sidelined.
Energy-efficient AI solutions
Sustainability will be a key future development. Aviation companies are increasingly focusing on reducing their carbon footprint, and AI algorithms will play a critical role. These algorithms can optimize warehouse layouts to reduce energy consumption or analyze transportation routes to minimize emissions during part shipments.
Predictive collaboration with regulators
AI systems of the future will also include predictive compliance tools that engage with regulatory authorities proactively. By anticipating regulatory updates or new certification requirements, these systems will help aviation companies adapt inventory strategies most efficiently for uninterrupted operations and smoother audits.
Partnering with ePlaneAI for inventory management solutions
ePlaneAI’s automated inventory management solutions provide aviation supply chain managers with the features they need for future-proof success. From predictive analytics to real-time tracking, our solutions are designed to optimize inventory, reduce costs, and ensure seamless operations.
Ready to take your inventory management to the next level? Contact ePlaneAI today to learn more about our cutting-edge solutions.